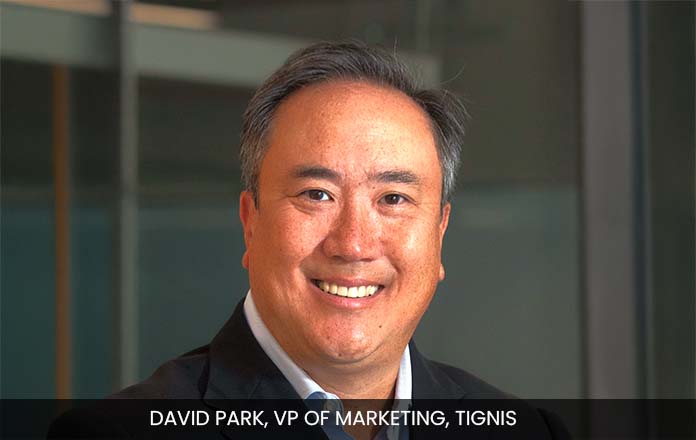
By David Park, VP of Marketing, Tignis
In today’s semiconductor industry, the discussion has shifted from “Should we adopt AI?” to “Are we ready for AI?” While some semiconductor manufacturing facilities (“fabs”) have made strides in AI implementation, many are still grappling with the AI-readiness gap—the difference between having raw data and having AI-ready data that can be effectively utilized to drive meaningful results.
Through numerous customer engagements, we have identified five key challenges that semiconductor fabs face when implementing AI solutions for their fab. These steps are vital for fabs to bridge the AI-readiness gap and fully unlock the enormous potential of AI and avoid frustration and unmet expectations.
Data Engineering – Laying the Foundation
AI thrives on data, but the reality is that most manufacturing data is not AI-ready. Most of the data that is collected is siloed, inconsistent, or lacks the necessary structure for automated and advanced analytics.
Fabs need to establish a solid data engineering process. This means defining the structure of the data, how it will be aggregated (and how often), and ensuring that the collected data is consistent over extended periods of time. A solid data engineering foundation will provide the necessary infrastructure for a successful AI implementation.
Without proper data engineering, manufacturing teams will spend weeks—if not months— wrangling their data after the AI tools are deployed, which delays meaningful analysis and creates the perception that AI is slow or ineffective.
Data Science – Unlocking the Power of Analytics
Once your manufacturing data is properly set up, the next challenge is ensuring that you have the right data science team in place. Historically, process engineers managed the data analysis in fabs, but as the complexity and volume of data have increased, there is a growing need for dedicated data scientists.
Data scientists may not always fully understand the physics and engineering involved in the manufacturing process, so it is important to have an environment that enables data scientists to collaborate with process engineers to quickly iterate and deliver actionable insights. Due to the complexity of analytics involved in semiconductor manufacturing, establishing an environment for collaboration will avoid the analytic bottlenecks that can slow down the AI analytic process.
Accessibility of Data – Breaking Down Silos
Another challenge many teams and individuals face is limited data access. Often, manufacturing data is stored behind layers of security or siloed within specific departments, limiting its availability for rapid analysis.
It is important that fabs have a plan for data democratization that makes data readily accessible to those who need it. Access privileges should be established in advance so that engineers are able to access relevant data on-demand to address real-time manufacturing issues and improve fab efficiency and reduce unplanned downtime.
Scalable Teams – Preparing for Growth
Fabs often rely on individuals to handle both data engineering and data science, which can limit scalability. As AI becomes more integrated into operations, the workload will increase, and relying on a single person to support both roles may result in a workload imbalance.
It is critical to set up your AI support teams that can scale as your AI adoption grows (and it will grow over time). Ideally, a plan will include separate data engineering and data science teams to handle the specific demands of AI within your organization. If that is not feasible, you may want to have a discussion with your solution provider to see if they are able to help in this area where needed.
Infrastructure – Preparing for the Future
Finally, fabs need to ensure their data infrastructure is capable of handling new AI requirements. This means not only collecting the right data but also ensuring that there are no blind spots. Many fabs discover too late that their sensors and instrumentation are not equipped to collect the types of data they need for their long-term analytic needs.
Assess your fab’s current data infrastructure and identify any gaps in coverage. While these gaps may not be critical in the short term, addressing them early on will ensure your fab is ready for more advanced AI analytics in the future.
Whether your fab is struggling with data collection, integration, or scaling your AI efforts, these five steps can help close the AI-readiness gap and ensure that AI implementation delivers the results you expect.
AI is More Than Just Tools – It is Also About Your AI-Readiness of Your Data
When considering an AI solution provider, you should have discussions around these five key areas in addition to discussing the actual AI products you want to evaluate for your manufacturing environment. If this is the first significant AI implementation for your fab, addressing these issues up front will help you achieve a much more successful outcome for your AI solution implementation.